NSDT工具推荐: Three.js AI纹理开发包 - YOLO合成数据生成器 - GLTF/GLB在线编辑 - 3D模型格式在线转换 - 可编程3D场景编辑器 - REVIT导出3D模型插件 - 3D模型语义搜索引擎 - AI模型在线查看 - Three.js虚拟轴心开发包 - 3D模型在线减面 - STL模型在线切割 - 3D道路快速建模
让我们通过微调 Llama 3.2 来找到一些精神上的平静。
我们需要安装 unsloth,以更小的尺寸实现 2 倍的快速训练
!pip install unsloth
!pip uninstall unsloth -y && pip install --upgrade --no-cache-dir "unsloth[colab-new] @ git+https://github.com/unslothai/unsloth.git"
我们将使用 Unsloth,因为它显著提高了微调大型语言模型 (LLM) 的效率,特别是 LLaMA 和 Mistral。使用 Unsloth,我们可以使用高级量化技术(例如 4 位和 16 位量化)来减少内存并加快训练和推理速度。这意味着我们甚至可以在资源有限的硬件上部署强大的模型,而不会影响性能。
此外,Unsloth 广泛的兼容性和定制选项允许执行量化过程以满足产品的特定需求。这种灵活性加上其将 VRAM 使用量减少高达 60% 的能力,使 Unsloth 成为 AI 工具包中必不可少的工具。它不仅仅是优化模型,而是让尖端 AI 更易于访问,更高效地应用于现实世界。
对于微调,我使用了以下设置:
- Torch 2.1.1 - CUDA 12.1 可实现高效计算。
- Unsloth 可实现大型语言模型 (LLM) 的 2 倍更快的训练速度。
- H100 NVL GPU 可满足密集处理要求,但你可以使用功率较低的 GPU,即 Kaggle GPU。
为什么是 LLaMA 3.2?
它是开源且可访问的,并提供了根据特定需求进行自定义和微调的灵活性。由于 Meta 的模型权重是开源的,因此可以非常轻松地对任何问题进行微调,我们将在 Hugging Face 的心理健康数据集上对其进行微调
1、Python库
数据处理和可视化
import os
import numpy as np
import pandas as pd
import seaborn as sns
import matplotlib.pyplot as plt
plt.style.use('ggplot')
LLM模型训练:
import torch
from trl import SFTTrainer
from transformers import TrainingArguments, TextStreamer
from unsloth.chat_templates import get_chat_template
from unsloth import FastLanguageModel
from datasets import Dataset
from unsloth import is_bfloat16_supported
# Saving model
from transformers import AutoTokenizer, AutoModelForSequenceClassification
# Warnings
import warnings
warnings.filterwarnings("ignore")
%matplotlib inline
2、调用数据集
data = pd.read_json("hf://datasets/Amod/mental_health_counseling_conversations/combined_dataset.json", lines=True)
3、探索性数据分析
让我们检查一下每个上下文中的单词长度:
data['Context_length'] = data['Context'].apply(len)
plt.figure(figsize=(10, 3))
sns.histplot(data['Context_length'], bins=50, kde=True)
plt.title('Distribution of Context Lengths')
plt.xlabel('Length of Context')
plt.ylabel('Frequency')
plt.show()
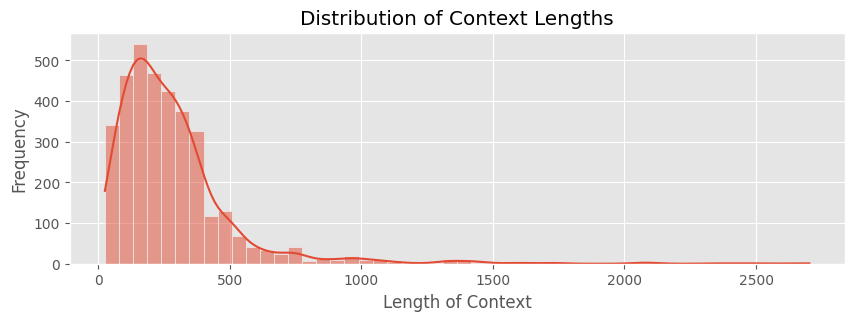
注意:如上所示,单词数最少为 1500 个,而且存在显著差异,因此我们只使用 1500 个或更少单词的数据。
filtered_data = data[data['Context_length'] <= 1500]
ln_Context = filtered_data['Context'].apply(len)
plt.figure(figsize=(10, 3))
sns.histplot(ln_Context, bins=50, kde=True)
plt.title('Distribution of Context Lengths')
plt.xlabel('Length of Context')
plt.ylabel('Frequency')
plt.show()
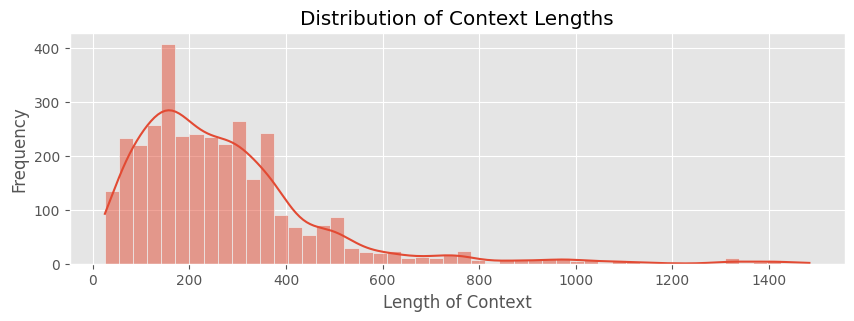
注意:现在可以使用这些数据。
现在让我们检查一下每个回复的单词长度:
ln_Response = filtered_data['Response'].apply(len)
plt.figure(figsize=(10, 3))
sns.histplot(ln_Response, bins=50, kde=True, color='teal')
plt.title('Distribution of Response Lengths')
plt.xlabel('Length of Response')
plt.ylabel('Frequency')
plt.show()
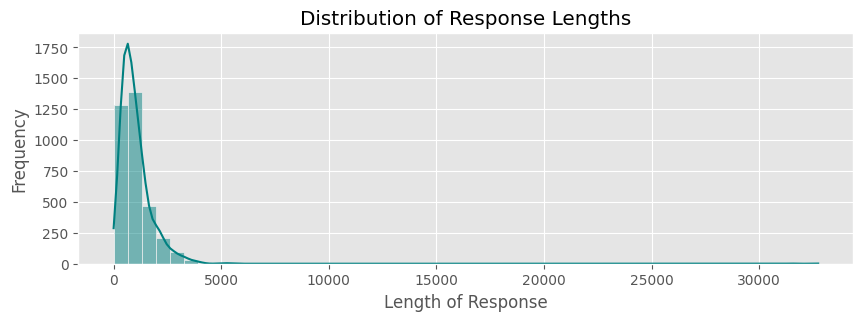
注意:这也是 4000 字长度的回应之后,出现了明显的下降。
filtered_data = filtered_data[ln_Response <= 4000]
ln_Response = filtered_data['Response'].apply(len)
plt.figure(figsize=(10, 3))
sns.histplot(ln_Response, bins=50, kde=True, color='teal')
plt.title('Distribution of Response Lengths')
plt.xlabel('Length of Response')
plt.ylabel('Frequency')
plt.show()
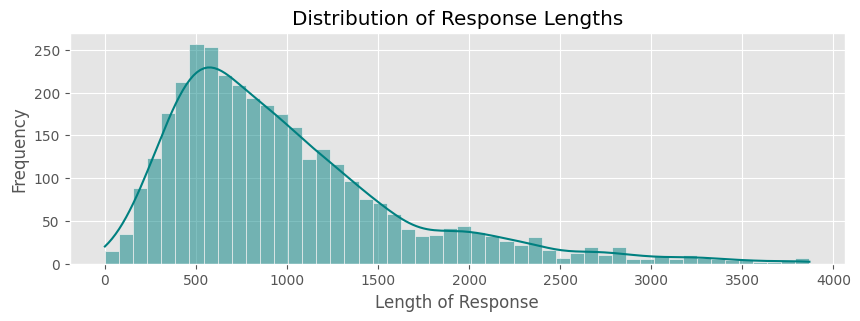
注意:不需要进行这样的数据准备来处理 LLM 模型的文本长度,但为了保持字数的一致性,我仅以 4000 个字以下的字为例,以便你可以根据需要进行任何数据预处理。
4、模型训练
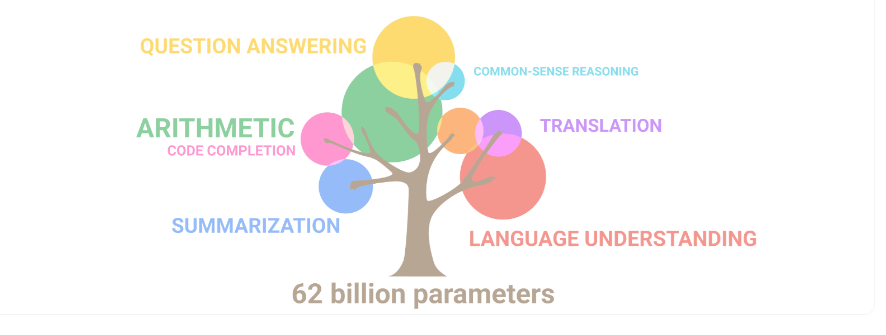
让我们深入研究 Llama 3.2 模型并在我们的数据上进行训练。
4.1 加载模型
我们将使用只有 10 亿个参数的 Llama 3.2,但你也可以使用 30 亿、110 亿或 900 亿个版本。
也可以根据你的要求遵循以下关键方面:
- 最大序列长度
我们使用了 max_seq_length
5020,这是模型中可以使用的最大标记数,可以在单个输入序列中处理。这对于需要处理长文本的任务至关重要,可确保模型在每次传递中都能捕获更多上下文。可以根据要求使用它。
- 加载 Llama 3.2 模型
使用 FastLanguageModel.from_pretrained
和特定的预训练模型 unsloth/Llama-3.2-1B-bnb-4bitt
加载模型和标记器。这针对 4 位精度进行了优化,可减少内存使用量并提高训练速度,而不会显着影响性能。 load_in_4bit=True
参数可实现这种高效的 4 位量化,使其更适合在性能较弱的硬件上进行微调。
- 应用 PEFT(参数高效微调)
然后我们使用 get_peft_model
配置模型,它应用了 LoRA(低秩自适应)技术。这种方法侧重于仅微调模型的特定层或部分,而不是整个网络,从而大大减少了所需的计算资源。
参数r=16
和 lora_alpha=16
等可调整这些自适应的复杂性和缩放比例。使用 target_modules
指定应调整模型的哪些层,其中包括涉及注意机制的关键组件,如 q_proj
、 k_proj
和 v_proj
。
use_rslora=True
可激活 Rank-Stabilized LoRA
,从而提高微调过程的稳定性。 use_gradient_checkpointing="unsloth"
确保通过选择性地仅存储必要的计算来优化训练期间的内存使用,从而进一步提高模型的效率。
- 验证可训练参数
最后,我们使用 model.print_trainable_parameters()
打印出将在微调期间更新的参数数量,从而验证是否只训练了模型的预期部分。
这种技术组合不仅使微调过程更加高效,而且更易于访问,即使在计算资源有限的情况下,你也可以部署此模型。
将 tokenz 的最大长度设置为 5020 足以作为低秩自适应 (LoRA) 进行训练,但您可以根据你的数据和要求使用。
max_seq_length = 5020
model, tokenizer = FastLanguageModel.from_pretrained(
model_name="unsloth/Llama-3.2-1B-bnb-4bit",
max_seq_length=max_seq_length,
load_in_4bit=True,
dtype=None,
)
model = FastLanguageModel.get_peft_model(
model,
r=16,
lora_alpha=16,
lora_dropout=0,
target_modules=["q_proj", "k_proj", "v_proj", "up_proj", "down_proj", "o_proj", "gate_proj"],
use_rslora=True,
use_gradient_checkpointing="unsloth",
random_state = 32,
loftq_config = None,
)
print(model.print_trainable_parameters())
4.2 为模型提要准备数据
现在是时候设计用于心理健康分析的格式提示了。此功能从心理学角度分析输入文本,识别情绪困扰、应对机制或整体心理健康的指标。它还强调潜在的担忧或积极方面,为每个观察结果提供简要解释。我们将准备这些数据以供模型进一步处理,确保每个输入输出对都具有清晰的格式,以便进行有效分析。
要记住的要点:
- 数据提示结构
data_prompt
是一个格式化的字符串模板,旨在指导模型分析提供的文本。它包括输入文本(上下文)和模型响应的占位符。该模板专门提示模型识别心理健康指标,使模型更容易微调心理健康相关任务。
- 序列结束标记
从标记器中检索 EOS_TOKEN
以表示每个文本序列的结束。此标记对于模型识别提示何时结束至关重要,有助于在训练或推理期间维护数据的结构。
- 格式化函数
formatting_prompt
用于获取一批示例并根据 data_prompt
对其进行格式化。它遍历输入和输出对,将它们插入模板并在末尾附加 EOS 标记。然后,该函数返回一个包含格式化文本的字典,可用于模型训练或评估。
- 函数输出
该函数输出一个字典,其中键为“文本”,值是格式化字符串的列表。每个字符串代表模型的完整准备提示,结合了上下文、响应和结构化提示模板。
data_prompt = """Analyze the provided text from a mental health perspective. Identify any indicators of emotional distress, coping mechanisms, or psychological well-being. Highlight any potential concerns or positive aspects related to mental health, and provide a brief explanation for each observation.
### Input:
{}
### Response:
{}"""
EOS_TOKEN = tokenizer.eos_token
def formatting_prompt(examples):
inputs = examples["Context"]
outputs = examples["Response"]
texts = []
for input_, output in zip(inputs, outputs):
text = data_prompt.format(input_, output) + EOS_TOKEN
texts.append(text)
return { "text" : texts, }
4.3 格式化数据以进行训练
training_data = Dataset.from_pandas(filtered_data)
training_data = training_data.map(formatting_prompt, batched=True)
4.4 使用自定义参数和数据进行模型训练
使用 sudo apt-get update
刷新可用软件包列表,使用 sudo apt-get install build-essential
安装必备工具。如果出现任何错误,请在 shell 上运行此命令。
#sudo apt-get update
#sudo apt-get install build-essential
4.5 训练设置开始微调!
我们将使用模型和标记器以及训练数据集初始化 SFTTrainer
。 dataset_text_field
参数指定数据集中包含我们上面准备的用于训练的文本的字段。训练器负责管理微调过程,包括数据处理和模型更新。
训练参数如下:
TrainingArguments
类用于定义训练过程的关键超参数。这些包括:
learning_rate=3e-4
:设置优化器的学习率。per_device_train_batch_size=32
:定义每个设备的批次大小,优化 GPU 使用率。num_train_epochs=20
:指定训练周期数。fp16=not is_bfloat16_supported()
和bf16=is_bfloat16_supported()
:启用混合精度训练以减少内存使用量,具体取决于硬件支持。optim="adamw_8bit"
:使用 8 位 AdamW 优化器来高效使用内存。weight_decay=0.01
:应用权重衰减以防止过度拟合。output_dir="output"
:指定将保存训练模型和日志的目录。
最后,我们调用 trainer.train()
方法来启动训练过程。它使用我们定义的参数来微调模型,调整权重并从提供的数据集中学习。训练器还处理数据打包和梯度累积,优化训练管道以获得更好的性能。
有时 pytorch 会保留内存并且不会释放回来。设置此环境变量可以帮助避免内存碎片。你可以在运行模型之前在环境或脚本中设置它
export PYTORCH_CUDA_ALLOC_CONF=expandable_segments:True
如果 GPU 中不再需要变量,可以使用 del 删除它们,然后调用
torch.cuda.empty_cache()
trainer=SFTTrainer(
model=model,
tokenizer=tokenizer,
train_dataset=training_data,
dataset_text_field="text",
max_seq_length=max_seq_length,
dataset_num_proc=2,
packing=True,
args=TrainingArguments(
learning_rate=3e-4,
lr_scheduler_type="linear",
per_device_train_batch_size=16,
gradient_accumulation_steps=8,
num_train_epochs=40,
fp16=not is_bfloat16_supported(),
bf16=is_bfloat16_supported(),
logging_steps=1,
optim="adamw_8bit",
weight_decay=0.01,
warmup_steps=10,
output_dir="output",
seed=0,
),
)
trainer.train()
4.6 推理
text="I'm going through some things with my feelings and myself. I barely sleep and I do nothing but think about how I'm worthless and how I shouldn't be here. I've never tried or contemplated suicide. I've always wanted to fix my issues, but I never get around to it. How can I change my feeling of being worthless to everyone?"
注意:让我们使用微调模型进行推理,以便根据与心理健康相关的提示生成反应!
以下是需要注意的一些要点:
model = FastLanguageModel.for_inference(model)
专门为推理配置模型,优化其生成响应的性能。
使用 tokenizer
对输入文本进行标记,它将文本转换为模型可以处理的格式。我们使用 data_prompt
来格式化输入文本,而将响应占位符留空以从模型获取响应。 return_tensors = "pt"
参数指定输出应为 PyTorch 张量,然后使用 .to("cuda")
将其移动到 GPU 以加快处理速度。
model.generate
方法根据标记化的输入生成响应。参数 max_new_tokens = 5020
和 use_cache = True
确保模型可以通过利用来自先前层的缓存计算来有效地生成长而连贯的响应。
model = FastLanguageModel.for_inference(model)
inputs = tokenizer(
[
data_prompt.format(
#instructions
text,
#answer
"",
)
], return_tensors = "pt").to("cuda")
outputs = model.generate(**inputs, max_new_tokens = 5020, use_cache = True)
answer=tokenizer.batch_decode(outputs)
answer = answer[0].split("### Response:")[-1]
print("Answer of the question is:", answer)
问题的答案如下:
I'm sorry to hear that you are feeling so overwhelmed. It sounds like you are trying to figure out what is going on with you. I would suggest that you see a therapist who specializes in working with people who are struggling with depression. Depression is a common issue that people struggle with. It is important to address the issue of depression in order to improve your quality of life. Depression can lead to other issues such as anxiety, hopelessness, and loss of pleasure in activities. Depression can also lead to thoughts of suicide. If you are thinking of suicide, please call 911 or go to the nearest hospital emergency department. If you are not thinking of suicide, but you are feeling overwhelmed, please call 800-273-8255. This number is free and confidential and you can talk to someone about anything. You can also go to www.suicidepreventionlifeline.org to find a local suicide prevention hotline.<|end_of_text|>
注意:以下是我们如何安全地将经过微调的模型及其标记器推送到 Hugging Face Hub,以便任何人都可以使用: ImranzamanML/1B_finetuned_llama3.2 。
os.environ["HF_TOKEN"] = "hugging face token key, you can create from your HF account."
model.push_to_hub("ImranzamanML/1B_finetuned_llama3.2", use_auth_token=os.getenv("HF_TOKEN"))
tokenizer.push_to_hub("ImranzamanML/1B_finetuned_llama3.2", use_auth_token=os.getenv("HF_TOKEN"))
注意:我们还可以在机器本地保存微调后的模型及其标记器。
model.save_pretrained("model/1B_finetuned_llama3.2")
tokenizer.save_pretrained("model/1B_finetuned_llama3.2")
下面的代码展示了如何加载已保存的模型并使用它!
model, tokenizer = FastLanguageModel.from_pretrained(
model_name = "model/1B_finetuned_llama3.2",
max_seq_length = 5020,
dtype = None,
load_in_4bit = True)
原文链接:Fine-Tuning 1B LLaMA 3.2: A Comprehensive Step-by-Step Guide with Code
BimAnt翻译整理,转载请标明出处